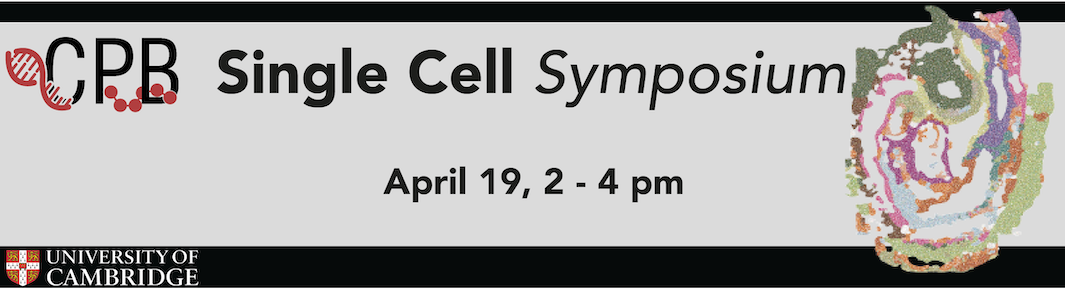
CPB Single Cell Symposium
April 19, 2-4 pm
An afternoon of discussion around cutting-edge methods and applications of single-cell genomics
Scientific organizer - John Marioni
Registration
This event is restricted to members of the University of Cambridge or partner institutions (the Animal Health Trust, Babraham Institute, British Antarctic Survey, Cambridge Crystallographic Data Centre, European Bioinformatics Institute, MRC Laboratory of Molecular Biology, National Institute of Agricultural Botany and the Wellcome Sanger Institute). Please use your institutional email address to register.
Programme (BST time)
Abstracts: scroll down or click the speakers names
14:00 – 14:10 – John Marioni Opening and Single cell approaches overview
EMBL-EBI and CRUK Cambridge Institute, UK
14:10 – 14:50 – Rahul Satija Integrated analysis of single-cell data across modalities and technologies
New York Genome Center, USA
14:50 – 15:00 – Break
15:00 – 15:20 – Emma Dann Milo: differential abundance testing on single-cell data using KNN graphs
Wellcome Sanger Institute, UK
15:20 – 15:40 – Naomi Moris Mapping space and time in developing in vitro model systems
Francis Crick Institute, UK
John Marioni EMBL-EBI and CRUK Cambridge Institute, UK
www.ebi.ac.uk/research/marioni
Single cell approaches overview
A cell’s transcriptional signature provides key insight into its identity and function. Within an organism, different tissues possess distinct gene expression profiles, and distinct profiles are also seen during normal development. Mutations that alter these expression profiles have been associated with adverse phenotypes ranging from a delayed immune response through to diseases including cancer.
Until relatively recently, molecular fingerprints were generated by profiling gene expression levels from bulk populations of millions of input cells. The expression value for each gene was therefore an average of its expression across the whole population of input cells. However, there are many biological questions where bulk measures of gene expression are insufficient. During early development, for example, each individual cell can have a distinct function and role. Moreover, many cancers are polyclonal, where each clone has a distinct expression profile; these clones are typically difficult to dissect experimentally. In these settings, the cellular ecosystem can only be properly understood by profiling molecular features at single cell resolution.
The past decade has seen a revolution in single-cell transcriptomics. In this introduction, I will describe some of the advances made and how assaying gene expression and other molecular features at the single-cell level represents a powerful tool for biological discovery.
Rahul Satija New York Genome Center - NYU
Integrated analysis of single-cell data across modalities and technologies
Emma Dann Wellcome Sanger Institute
www.ebi.ac.uk/research/marioni
Milo: differential abundance testing on single-cell data using KNN graphs
Single-cell omic protocols applied to disease, development or mechanistic studies can reveal the emergence of aberrant cell states or changes in differentiation. These perturbations can manifest as a shift in the abundance of cells associated with a biological condition. Current computational workflows for comparative analyses typically use discrete clusters as input when testing for differential abundance between experimental conditions. However, clusters are not always an optimal representation of the biological manifold on which cells lie, especially in the context of continuous differentiation trajectories. To overcome these barriers to discovery, we have developed Milo, a flexible and scalable statistical framework that performs differential abundance testing by assigning cells to partially overlapping neighbourhoods on a k-nearest neighbour graph. Our method samples and refines neighbourhoods across the graph and leverages the flexibility of generalized linear models, making it applicable to a wide range of experimental settings. With extensive benchmarking on real and simulated KNN graphs, we show that Milo is both robust and sensitive, and can reveal subtle but important cell state perturbations that are obscured by discretizing cells into clusters. We illustrate the power of Milo by identifying the perturbed differentiation during aging of a lineage-biased thymic epithelial precursor state and by uncovering extensive perturbation to multiple lineages in human cirrhotic liver.
Naomi Moris The Francis Crick Institute
Mapping space and time in developing in vitro model systems
During development, sequential cell fate decisions generate diversity by carefully orchestrating their organisation across space and time. Often the dynamics of cell fate decisions directly relate to spatial location, such as in early development where decisions are organised along the embryonic anterior-posterior axis. Advances in single-cell transcriptomics have enabled the characterisation of numerous cell states to be identified with high-resolution, yet these methods typically require cellular dissociation which disrupts such spatial information. In silico techniques, such as pseudotime approaches, often aim to ‘reintroduce’ dynamics by using information inherent in the data but make several assumptions. Instead, we have sought to identify spatial information inherent in such single-cell datasets using a parallel approaches of spatial-transcriptomic sequencing and complementary single-cell data. This approach allows us to try to reconstruct cellular arrangements that closely reflect the original organisation of source tissues with broader implications where preserving spatial information in high-resolution data could allow the identification of novel spatial and dynamic trends along embryonic axes.