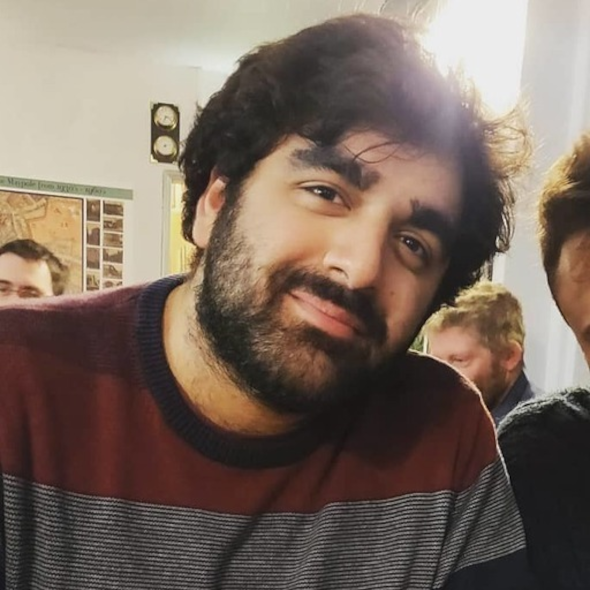
Submitted by Paulina Urriola... on Mon, 14/10/2024 - 11:22
Quantitative microbiology with widefield microscopy: navigating optical artefacts for accurate interpretations
Georgeos Hardo, Ruizhe Li & Somenath Bakshi, npj Imaging volume 2, Article number: 26 (2024) | doi: https://doi.org/10.1038/s44303-024-00024-4
By Georgeos Hardo, Department of Engineering, University of Cambridge, Cambridge, UK
1. How would you explain the main findings of your paper in layman's terms?
We explored how small microbial cells, particularly bacteria, create significant challenges when imaged using widefield fluorescence microscopy. Due to their small size, microbial cells approach the physical limits of what microscopes can clearly resolve. This results in blurry and distorted images that make it difficult to accurately measure properties such as cell size, shape, fluorescence intensity, and even the number of molecules within each cell. Unfortunately, this corruption means that one has no access to any ground truth information about their images, meaning correction and calibration is extremely difficult. To address these challenges, we developed a virtual microscopy platform, capable of simulating microscope images of microbial cells under various conditions. These simulated images are accompanied by perfect ground truth, and thus we were able to systematically investigate these limitations and identify specific errors that arise from the interaction between projection and diffraction effects. These errors significantly impact the accuracy analysing of bioimaging data, leading us to propose improved experimental conditions, imaging techniques, and machine learning-based methods to mitigate these issues and provide more reliable data for researchers.
- Miscalculation of cell intensity within microcolonies: When bacterial cells are densely packed in microcolonies, light from one cell bleeds into neighbouring cells due to diffraction, leading to an overestimation of their fluorescence intensity. This artifact, known as "light bleedthrough," causes cells in the centre of a colony to appear brighter than they actually are, while isolated cells or those at the colony's edges appear dimmer. As a result, the true intensity of individual cells is distorted by their neighbours. We find that the intensity of a single cell can be artificially inflated by up to 2-3 times their true value, complicating efforts to accurately measure gene expression levels or protein abundance within cells and across populations of cells. We propose the use of microfluidic devices (known as the mother machine), where cells can be maintained at a specific distance to one another to eliminate/mitigate this effect.
- Miscalculation of cell size due to fluorescence effects:While widefield imaging of single cells is often used for estimating cell size, the effects of projection and diffraction are rarely accounted for. Fluorescence imaging introduces errors in estimating cell size, particularly for bacterial cells, where projection and diffraction effects combine to distort the images. For cells stained with fluorescent dyes that mark the membrane, the light diffracts and shifts toward the center of the cell in 2D images. This results in an underestimation of cell width—by as much as 20-40% for smaller bacterial cells—because membrane fluorescence is incorrectly interpreted. Similarly, cells with uniformly distributed fluorescent markers inside the cytoplasm exhibit an overestimation of size due to diffraction spreading the signal beyond the true cell boundary. These biases are influenced by factors such as the imaging wavelength, the depth of the field, and the choice of fluorescence marker. The use of synthetic data to train deep learning models can help to virtually eliminate these errors during analysis.
- Miscounting of single molecules:In fluorescence microscopy, counting single molecules, such as proteins or mRNA, is challenging due to diffraction and projection effects. When molecules are too close together within a cell (in 3D), they can overlap in the 2D image and appear as a single, larger blurry spot, making it impossible to resolve individual molecules. This leads to undercounting, especially when cells have low copy numbers of tagged molecules. Our simulations showed that even with only 5-15 molecules per cell, counting errors can reach 40%, with the molecules often merging into one visible spot. Additionally, molecules located out of focus (in the Z-plane) are often too dim to detect, further contributing to undercounting. Once again, combining microfluidic techniques with deep learning models trained using synthetic data can push single molecule counting accuracies to previously unrealisable levels.
2. How does this paper contribute to cross-disciplinary research in physical biology?
Because the artefacts discussed are a function of imaging microbes under specific conditions, the solutions draw upon methods from microfluidics, microscopy, and deep learning, bringing together microbiologists, engineers, microscopists, and bioimage analysts. The understanding of the physics of image formation is critical will help microbiologists avoid errors that could previously distort their results by changing experimental design and using appropriate analysis platforms. Good quantitative microbiology is therefore necessarily cross-disciplinary in order to generate new biological insights.
3. Can you share a fun or interesting fact about the paper or the project that led to it?
This paper has been in the works, on and off, for approximately 3 years. It's the combination of many findings we had observed by chance. We knew these artefacts existed but didn't know to what extent, and how devastating their effects could be to experimental analysis. One of the reasons we stumbled across this was due to simple agar pad experiments, where single bacterial cells are scattered across an agar pad at low density for imaging. If cells are at too high of a density, one typically sees that these cells cluster into “preformed” colonies. These colonies have not arisen from a single cell, rather just from the fact that cells were in very close proximity upon being imaged. Typically we throw such data away, since we’re interested in growth arising from a single cell, however we realised (in very low noise fluorescent gene expression) that we could observe predictable and repeatable spatial heterogeneity in these preformed colonies, despite there being no time for any biological heterogeneity to creep in via growth. This spatial heterogeneity necessarily comes from the imaging system, and not a biological phenomenon (image attached).
It was at a CPB funded SuperBug meetup event when discussions made us realise we could actually probe these artefacts. We had previously published our virtual microscopy platform (SyMBac: Synthetic Micrographs of Bacteria), which was originally intended to generate synthetic training data for bioimage analysis deep learning models. We realised this platform could also be used to simulate microscopy data and artificially control the strength of these imaging artefacts, allowing us to learn about the errors creeping into our image analysis results.
Would you like to be featured in future Author Interviews? Just get in touch at admin@physbiol.cam.ac.uk.